In the high-stakes game of talent retention, organizations are no longer relying on gut feelings or exit interviews. The new frontier of workforce management is predictive analytics—a sophisticated approach that uses machine learning to identify potential flight risks before they become resignation letters.
The Hidden Cost of Turnover
Let’s start with a stark reality: employee turnover is expensive. According to Deloitte research, the total cost of losing an employee can range from 50% to 250% of their annual salary. In a competitive job market, prevention isn’t just better than cure—it’s a strategic imperative.
How Predictive Analytics Works: Decoding Employee Behavior
The Data Alchemy
Modern predictive analytics goes far beyond traditional HR metrics. It’s a complex fusion of:
- Performance data
- Engagement survey results
- Communication patterns
- Career progression indicators
- External market signals
- Personal sentiment analysis
Machine Learning Models: The Early Warning System
Sentiment Analysis Algorithms
Advanced natural language processing (NLP) tools now analyze:
- Email tone and communication frequency
- Internal chat platform interactions
- Performance review language
- Social media professional sentiment
Behavioral Pattern Recognition
Machine learning models can now detect subtle shifts in:
- Reduced collaboration
- Decreased meeting participation
- Changes in work output quality
- Subtle communication pattern changes
Real-World Implementation Strategies
Case Study: Microsoft’s Retention Intelligence
Microsoft developed an internal predictive retention model that:
- Analyzed over 30 different data points per employee
- Achieved 85% accuracy in identifying potential departures
- Reduced unplanned turnover by 22% in two years
Google’s People Analytics Approach
Google’s method involves:
- Continuous engagement pulse surveys
- Real-time sentiment tracking
- Proactive career development interventions
- Personalized retention strategies
Ethical Considerations and Challenges
Privacy Paradox
While predictive analytics offers powerful insights, it raises critical ethical questions:
- Transparency in data collection
- Employee consent
- Preventing algorithmic bias
- Maintaining human-centric approach
Balancing Technology and Human Touch
The key is using predictive analytics as an indicator, not a definitive judgment. Successful implementations combine:
- Machine-generated insights
- Human empathy
- Personalized intervention strategies
Cutting-Edge Technologies Powering Retention Analytics
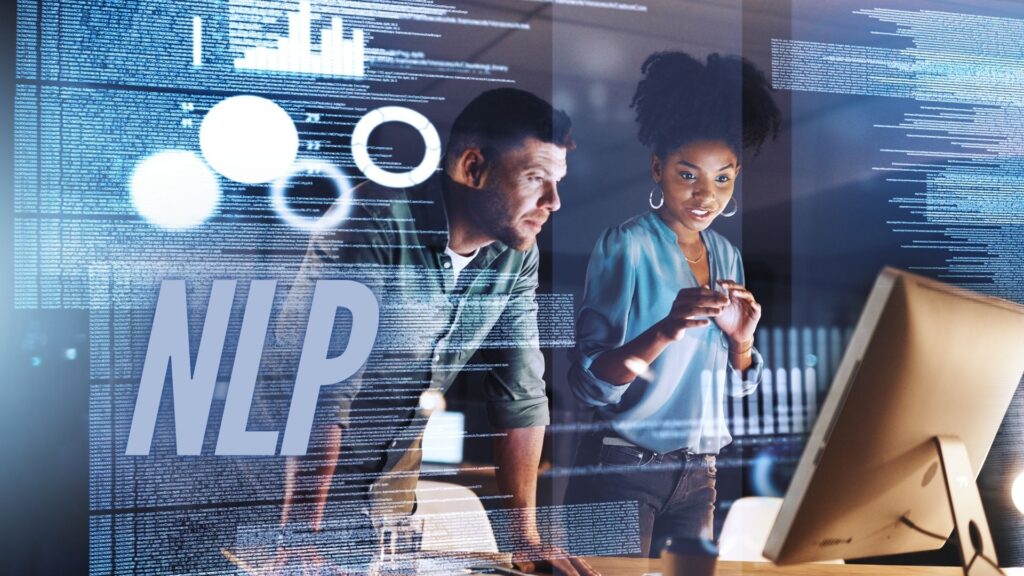
1. Natural Language Processing (NLP)
- Analyzing communication sentiment
- Detecting stress indicators
- Identifying potential disengagement signals
2. Network Analysis Algorithms
- Mapping internal collaboration networks
- Identifying isolation risks
- Understanding team dynamics
3. Reinforcement Learning Models
- Dynamic adjustment of retention strategies
- Personalized intervention recommendations
- Continuous model improvement
Practical Implementation Framework
Data Collection Strategy
- Holistic Data Gathering
- Performance management systems
- Learning and development platforms
- Communication tools
- External market signals
- Advanced Signal Integration
- Combine structured and unstructured data
- Use machine learning for pattern recognition
- Create dynamic employee profiles
- Intervention Design
- Personalized career development plans
- Targeted engagement initiatives
- Proactive mentorship programs
Emerging Trends and Future Outlook
AI-Driven Career Pathing
- Predictive skills mapping
- Internal mobility recommendations
- Personalized learning journeys
Emotional Intelligence Integration
- Advanced sentiment analysis
- Psychological safety metrics
- Holistic well-being tracking
Quantifiable Benefits
Potential Impact
- 30-50% reduction in unexpected turnover
- 25% improvement in internal mobility
- Significant cost savings in recruitment
- Enhanced organizational agility
Challenges in Adoption
- Data Quality
- Require comprehensive, clean data
- Need for continuous data refinement
- Technological Investment
- Significant initial infrastructure costs
- Continuous model training
- Cultural Transformation
- Shift from reactive to predictive mindset
- Building trust in data-driven approaches
Action Steps for Organizations
- Assessment
- Audit current retention strategies
- Evaluate data collection capabilities
- Technology Investment
- Select appropriate predictive analytics tools
- Build cross-functional analytics teams
- Pilot Programs
- Start with controlled, small-scale implementations
- Measure and iterate
- Continuous Learning
- Regular model retraining
- Stay updated with technological advancements
The Human-AI Partnership
The future of retention isn’t about replacing human intuition but augmenting it. Predictive analytics provides the map, but empathetic leadership charts the course.
Organizations that master this delicate balance—leveraging machine learning’s predictive power while maintaining human connection—will emerge as talent magnets in an increasingly competitive landscape.
The retention revolution is here. Are you ready to predict the future of your workforce?